Introduction about Machine Learning
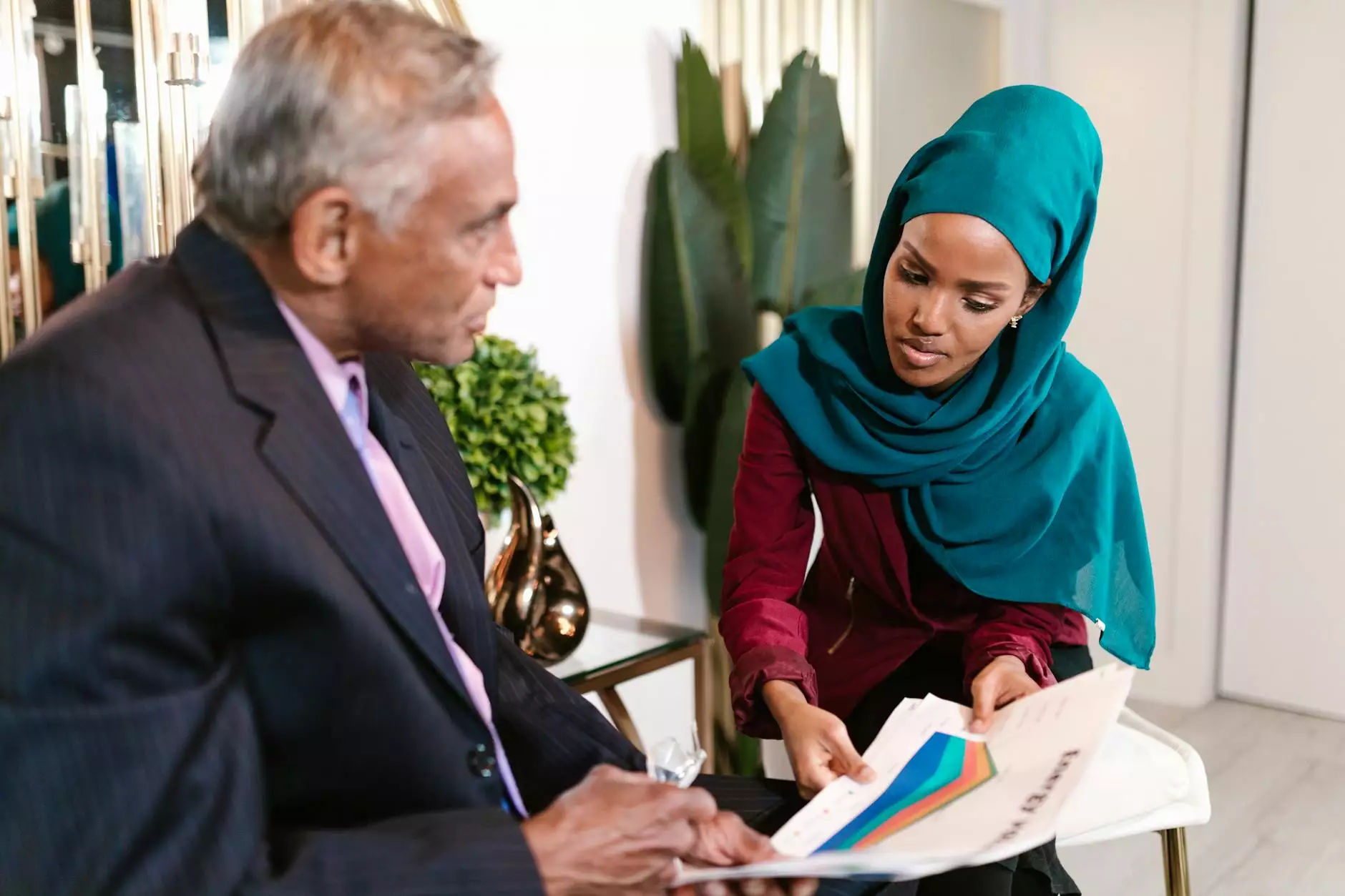
In today's rapidly evolving digital landscape, machine learning has emerged as a pivotal technology that empowers businesses to harness data and drive innovation. As part of the broader field of artificial intelligence (AI), machine learning enables systems to learn from data, improving their performance over time without explicit programming. This article serves as a detailed introduction about machine learning, exploring its fundamental concepts, practical applications, and transformative impact on various industries.
What is Machine Learning?
Machine learning is a subset of AI that focuses on building systems that can learn from and make predictions based on data. While traditional programming involves writing a set of rules for the computer to follow, machine learning leverages algorithms that enable systems to identify patterns and make decisions independently.
At its core, machine learning revolves around the idea that systems can automatically improve their performance with experience. This is achieved through two primary processes:
- Training: The system is exposed to a vast dataset from which it learns.
- Inference: Once trained, the system can make predictions or decisions based on new, unseen data.
Types of Machine Learning
Machine learning encompasses a variety of approaches, each tailored to specific types of data and desired outcomes. The main types of machine learning include:
1. Supervised Learning
In supervised learning, models are trained on labeled data, which means that both input data and the correct output are provided. This approach is commonly used for applications such as:
- Email spam detection
- Image recognition
- Predictive analytics in finance
2. Unsupervised Learning
Conversely, unsupervised learning involves training models on data without explicit labels. The system attempts to find hidden patterns or intrinsic structures within the data. Common applications include:
- Customer segmentation in marketing
- Anomaly detection in network security
- Recommendation systems
3. Reinforcement Learning
Reinforcement learning is inspired by behavioral psychology, where agents learn by receiving rewards or penalties based on their actions in an environment. This type of learning is crucial for applications like:
- Game AI
- Robotics
- Self-driving cars
The Importance of Machine Learning in Business
Incorporating machine learning into business strategies can yield significant advantages, including:
1. Enhanced Decision-Making
By analyzing vast amounts of data and predicting outcomes, machine learning provides valuable insights that help businesses make informed decisions. Whether in sales forecasting or operational optimizations, data-driven decisions often outperform intuition-based choices.
2. Improved Customer Experience
Machine learning algorithms enable organizations to personalize customer interactions. From recommending products based on past purchases to tailoring marketing messages, businesses can create a more engaging customer experience that drives loyalty.
3. Cost Efficiency
By automating repetitive tasks and streamlining processes, machine learning can significantly reduce operational costs. For instance, predictive maintenance models can foresee equipment failures, thus minimizing downtime and repair expenses.
Applications of Machine Learning Across Industries
The versatility of machine learning allows it to be applied across diverse sectors. Here, we highlight several industries where machine learning is making a remarkable impact:
1. Healthcare
In the healthcare domain, machine learning is revolutionizing diagnostics and treatment. Machine learning algorithms can analyze medical images for early detection of diseases, predict patient outcomes, and personalize treatment plans. For instance, tools that evaluate x-rays can assist radiologists in diagnosing conditions more accurately and swiftly.
2. Finance
Financial institutions leverage machine learning to detect fraudulent transactions, assess credit risk, and automate trading strategies. By analyzing transaction patterns, algorithms can identify anomalies indicative of fraud, thereby enhancing security.
3. Retail
In retail, machine learning enhances inventory management, optimizes pricing strategies, and improves supply chain logistics. Predictive analytics can forecast demand for products, helping businesses maintain optimal inventory levels and reduce waste.
4. Manufacturing
Manufacturers utilize machine learning for quality control, predictive maintenance, and production optimization. By analyzing machine data, manufacturers can predict failures before they occur and reduce production costs by streamlining operations.
Challenges in Implementing Machine Learning
Despite its benefits, businesses face several challenges when integrating machine learning into their operations:
1. Data Quality and Quantity
The performance of machine learning models heavily relies on the quality and quantity of data. Poor-quality data can lead to inaccurate predictions and wrong decisions. Hence, organizations must invest in high-quality data collection and cleaning processes.
2. Understanding and Trusting AI
Another challenge is the need for transparency in AI decision-making. Businesses must ensure that stakeholders understand how machine learning models operate and trust their outputs. This requires ongoing education and communication.
3. Talent Scarcity
There's a growing demand for skilled professionals proficient in machine learning. However, finding individuals with the right expertise can be challenging, leading to project delays and limited implementation capabilities.
The Future of Machine Learning
The future of machine learning is bright and brimming with opportunities. As technology continues to advance, we can expect:
1. Enhanced Algorithms
Ongoing research will yield more sophisticated algorithms capable of tackling complex problems, improving efficiency, and expanding the scope of machine learning applications.
2. Greater Integration with Business Processes
Machine learning will become increasingly integrated into business workflows, leading to a more data-driven culture where decisions are continuously informed by real-time analytics.
3. Ethical and Responsible AI
The importance of ethical considerations in AI and machine learning will gain prominence, with organizations focusing on fairness, transparency, and accountability in their AI applications to build trust with consumers and stakeholders.
Conclusion
In conclusion, machine learning represents a transformative force for businesses in various sectors. By understanding its fundamental principles, applications, and potential challenges, organizations can better leverage its capabilities. From enhancing customer experiences to optimizing operational processes, the advantages are profound. As we move forward, embracing machine learning will not only remain an option but will become essential for sustained growth and competitiveness in an increasingly data-driven world. For more insights and personalized consulting in machine learning, explore our services at machinelearningconsulting.net.